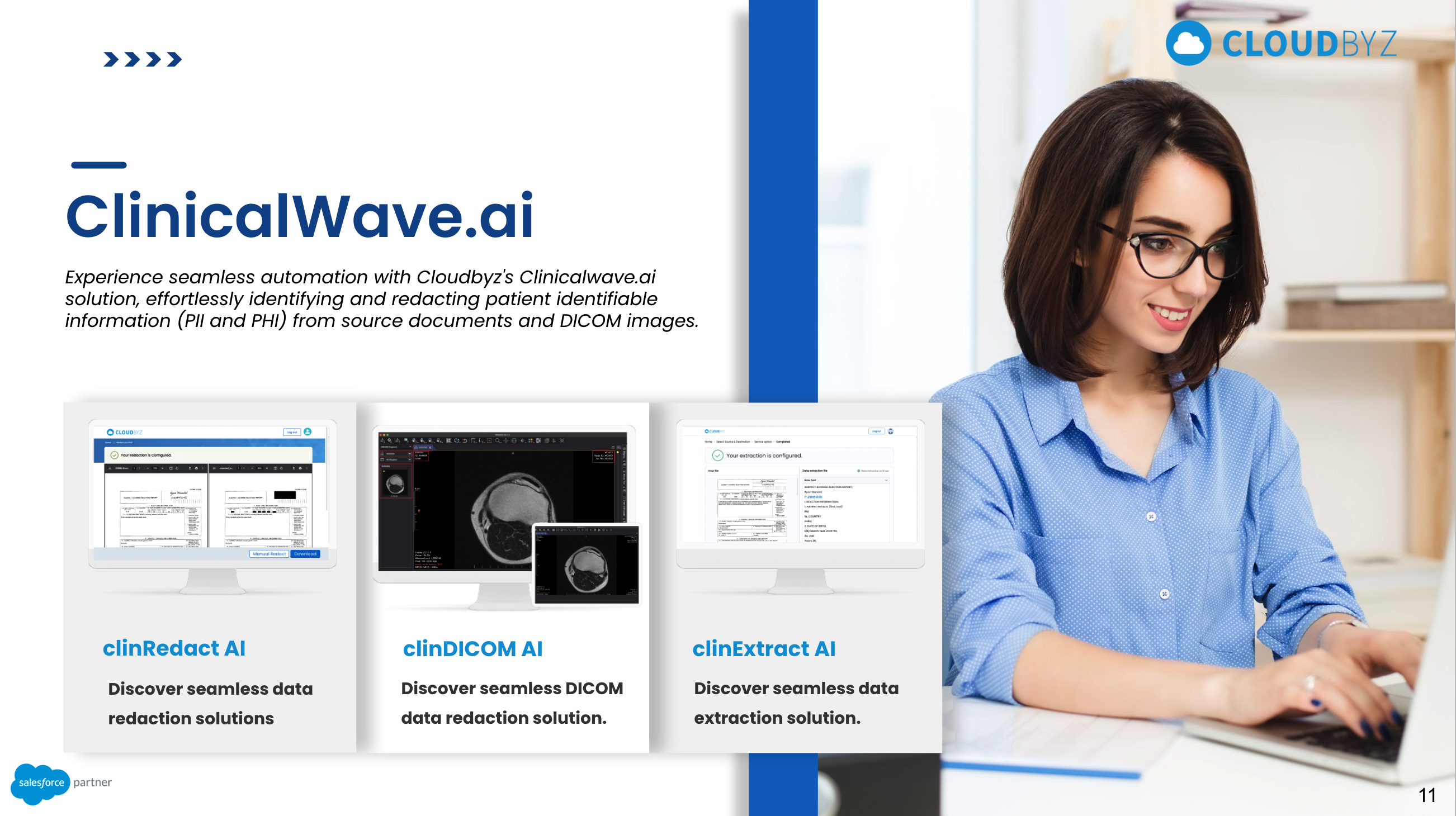
In the evolving landscape of clinical research, the integration of Artificial Intelligence (AI) presents a groundbreaking opportunity to enhance Clinical Data Management (CDM). As clinical trials become more complex and data volumes grow exponentially, AI’s potential to streamline processes, improve data accuracy, and accelerate study timelines is increasingly evident. This blog delves into how AI can revolutionize CDM and outlines key considerations for selecting the right AI solution.
The Role of AI in Clinical Data Management
1. Data Cleaning and Preprocessing
One of the most time-consuming aspects of CDM is ensuring that data is clean, consistent, and free from errors. AI algorithms can automate data cleaning processes, identifying and correcting discrepancies, missing values, and outliers with high precision. Machine learning models can be trained to recognize patterns and anomalies, reducing the need for manual intervention and significantly accelerating data preprocessing.
2. Real-Time Data Monitoring and Quality Control
AI can provide continuous, real-time monitoring of data quality throughout the clinical trial. Advanced algorithms can detect and flag potential issues as they arise, allowing for immediate corrective actions. This proactive approach to quality control helps maintain data integrity and ensures that high-quality data is available for analysis at all times.
3. Automated Data Entry and Management
Natural Language Processing (NLP) and Optical Character Recognition (OCR) technologies powered by AI can automate the extraction of data from various sources, such as clinical trial forms, electronic health records, and lab reports. This automation reduces manual data entry errors and accelerates data capture processes. AI-driven data management systems can also automate routine tasks, such as data migration and synchronization, further enhancing efficiency.
4. Advanced Data Analysis and Insights
AI can analyze large and complex datasets much faster and more accurately than traditional methods. Machine learning models can uncover hidden patterns, correlations, and trends that may not be apparent through manual analysis. These insights can lead to better decision-making, more accurate predictions of clinical outcomes, and the identification of potential biomarkers.
5. Predictive Analytics for Risk Management
AI can leverage historical and real-time data to predict potential risks and issues in clinical trials. Predictive analytics models can identify factors that may lead to patient dropouts, protocol deviations, or adverse events. By anticipating these risks, researchers can implement preventive measures, thereby enhancing study reliability and patient safety.
Important Considerations When Choosing an AI Solution for CDM
Selecting the right AI solution for clinical data management is crucial for maximizing its benefits. Here are some key considerations to keep in mind:
1. Regulatory Compliance
Ensure that the AI solution complies with relevant regulatory requirements, such as FDA 21 CFR Part 11, GDPR, and HIPAA. The solution should offer features like audit trails, electronic signatures, and data encryption to meet these standards. It’s essential to work with vendors who have a deep understanding of the regulatory landscape and can provide documentation and support for compliance.
2. Data Privacy and Security
Data privacy and security are paramount in clinical research. Choose an AI solution that implements robust security measures, including data encryption, secure access controls, and regular security audits. The solution should also offer data anonymization and de-identification capabilities to protect patient privacy.
3. Scalability and Flexibility
The AI solution should be scalable to handle large volumes of data and adaptable to the evolving needs of clinical trials. It should support integration with existing systems, such as Electronic Data Capture (EDC) systems, Clinical Trial Management Systems (CTMS), and electronic health records (EHR). Customizability is also crucial, allowing the solution to be tailored to specific study requirements and workflows.
4. Ease of Use and Implementation
A user-friendly interface and straightforward implementation process are critical for the successful adoption of AI in CDM. The solution should offer intuitive tools for data visualization, reporting, and analysis, enabling researchers to harness the power of AI without extensive training. Additionally, consider the vendor’s track record for customer support and their ability to provide ongoing training and resources.
5. Transparency and Explainability
AI models should be transparent and explainable, providing clear insights into how decisions and predictions are made. This is particularly important for gaining the trust of regulatory bodies and stakeholders. Look for solutions that offer detailed documentation, model interpretability features, and the ability to audit AI-driven processes.
6. Vendor Expertise and Experience
Partner with vendors who have a proven track record in clinical research and AI implementation. Assess their experience, client testimonials, and case studies to gauge their expertise. A vendor with deep industry knowledge can provide valuable insights and support throughout the implementation and operational phases.
Conclusion
AI has the potential to transform clinical data management, offering significant enhancements in data accuracy, efficiency, and insight generation. By automating routine tasks, enabling real-time monitoring, and providing advanced analytics, AI can accelerate clinical studies and improve overall data quality. However, selecting the right AI solution requires careful consideration of regulatory compliance, data security, scalability, ease of use, transparency, and vendor expertise.
As the clinical research landscape continues to evolve, embracing AI-driven CDM solutions will be essential for staying competitive and achieving success. By making informed decisions and leveraging the right AI technologies, organizations can unlock the full potential of their clinical data and drive better outcomes for patients and stakeholders alike.
Cloudbyz EDC is a powerful tool that enhances the quality, compliance, and efficiency of clinical trials. Its user-friendly interfaces, robust collaboration features, and comprehensive data security measures make it an ideal choice for organizations seeking to streamline their clinical research processes. By leveraging Cloudbyz EDC, researchers can ensure that their data is accurate, compliant, and secure, ultimately contributing to the success of their clinical trials.